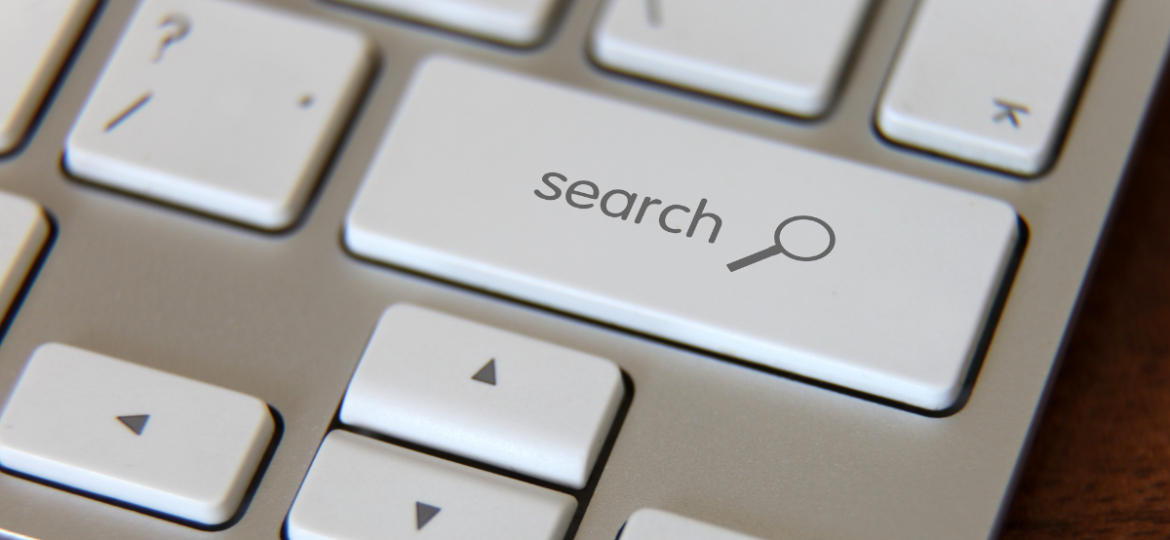
Finding precise information fast can mean the difference between staying ahead or falling behind. This is where semantic search comes into play, offering a sophisticated alternative to traditional keyword-based search methods. Unlike conventional searches that often bring up irrelevant results due to rigid keyword dependencies, semantic search understands the intent and contextual meaning behind queries. This advanced approach delivers significantly more relevant results, even when exact keywords are not used. In this article we emphasize the enhanced outcomes generated by AI-driven searches, highlighting the reasons why industries are progressively embracing this technology.
A. Transforming search with semantic search
Example 1
Consider the query “banks that went bankrupt.” Traditional search engines, using term frequency algorithms like BM25 might pull up a list of results containing the related terms. However, this doesn’t always answer the user’s underlying question about which banks have actually gone bankrupt. In this specific case you can see that a standard model like BM25 provides irrelevant answers. Here, ‘Banks’ is considered a surname and therefore, the search result provides a list of people named Banks. The search results do not include any real ‘banks’ that went bankrupt.
Semantic search, on the other hand, provides better results. It understands the broader context of “bankruptcy” in relation to “banks.” Therefore, it doesn’t just look for the keywords but interprets the meaning, bringing forward articles or data entries specifically about banks that have filed for bankruptcy, even if the phrasing in the documents isn’t an exact match to the search terms.
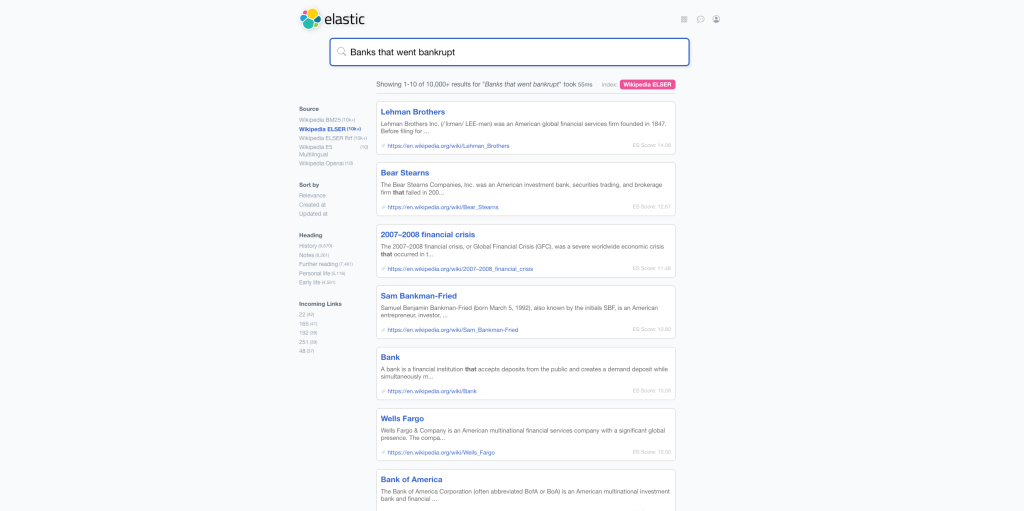
Example 2
How do you surface the best results, when the search query contains none of the keywords used in your search task? Another query, “how fast should my internet be,” showcases semantic search’s handles diverse expressions leading to the same inquiry. Traditional searches may direct you to general articles about internet speeds or technical specifications, which might not directly address your personal or business needs.
Using semantic search, the query processes not just the words but the intent behind them. It effectively matches the query to related terms like “required connection speed for streaming” or “internet speed recommendations for gaming,” offering targeted and highly relevant results. This is particularly useful for businesses looking to understand specific operational requirements or individuals trying to optimize their home setup.
Why businesses should care about semantic search
For business leaders, adopting semantic search technology means enhanced efficiency and improved satisfaction—both for internal stakeholders and customers. Internally, employees save time and frustration by quickly locating the exact information they need. Externally, customers enjoy a smoother, more intuitive interaction with your digital platforms, enhancing their overall experience and your brand reputation.
Moreover, as data continues to grow in volume and complexity, the ability to sift through and find the most pertinent information rapidly is becoming increasingly critical. Semantic search doesn’t just find keywords; it finds meanings, which is essential for making informed decisions faster.
B. Transforming search with image search
Take, for instance, the practical application within the fashion and retail industries. Raccoons developed a system in collaboration with Elk Factory where users could search using queries such as “blue t-shirt with small Nike logo” in the search bar. Instead of merely scanning for text matches, the search engine interprets the query to understand the meaning behind each element. The result? The search engine displays images of blue t-shirts with a small Nike logo, precisely matching the user’s request. This is possible because the engine uses advanced algorithms to analyze the attributes of clothing items stored in its database, such as color, brand logos, and design elements, connecting these features with the intent of the query.
This technology not only simplifies the user experience by allowing more natural language input but also enhances the accuracy of search results. Customers and employees alike can find exactly what they’re looking for with minimal effort, reducing search times and improving satisfaction.
The technical side: a Dual Approach to automating image tagging
For this demonstration, we employed Gemini to automate the tagging of 16,000 images. This approach included:
- Structured Descriptions: Gemini extracted precise attributes such as clothing type, color, and pattern.
- Textual Descriptions: It also generated natural language descriptions for each image.
- Data Integration: These descriptions were merged into a JSON file for each image, creating a comprehensive, searchable dataset stored in Elastic.
In the demo Raccoons used a dual search strategy to highlight the capabilities of Elastic and Gemini:
- Textual Embedding: Using Elastic’s ElserV2 model, we transformed textual descriptions into searchable embeddings, enhancing the granularity of search results.
- Structured Query Transformation: Gemini processed user queries into structured search fields, allowing for the retrieval of images based on specific attributes defined in the query.
This demo not only displayed how semantic search could refine search outcomes but also demonstrated its efficiency in handling large datasets with minimal human effort. By integrating advanced AI tools like Elastic and Gemini, we showed that businesses could unlock valuable data from extensive image libraries, providing richer customer experiences and streamlined operations.

the business impact of semantic image search
For businesses, the implications of such technology are profound. Retail companies can offer an enhanced online shopping experience, allowing customers to find products through highly specific descriptions. This capability can significantly improve conversion rates and customer loyalty as shoppers find exactly what they need quickly. Internally, companies can manage their inventories more effectively. Staff can locate items or check stock levels using straightforward queries, streamlining operations and inventory management.
c. conclusion: Embracing the future of search
The shift from keyword-focused to semantic and image search represents a significant step forward in information retrieval technology. For businesses ready to enhance their search capabilities, it promises not only to refine information discovery but also to transform how knowledge is accessed and utilized across industries.
As more companies recognize and implement the benefits of this technology, we can expect a surge in productivity and a sharper competitive edge. By understanding the intricacies and applications of semantic and image search, businesses can ensure they are not merely keeping up but leading the way in the information-driven economy.