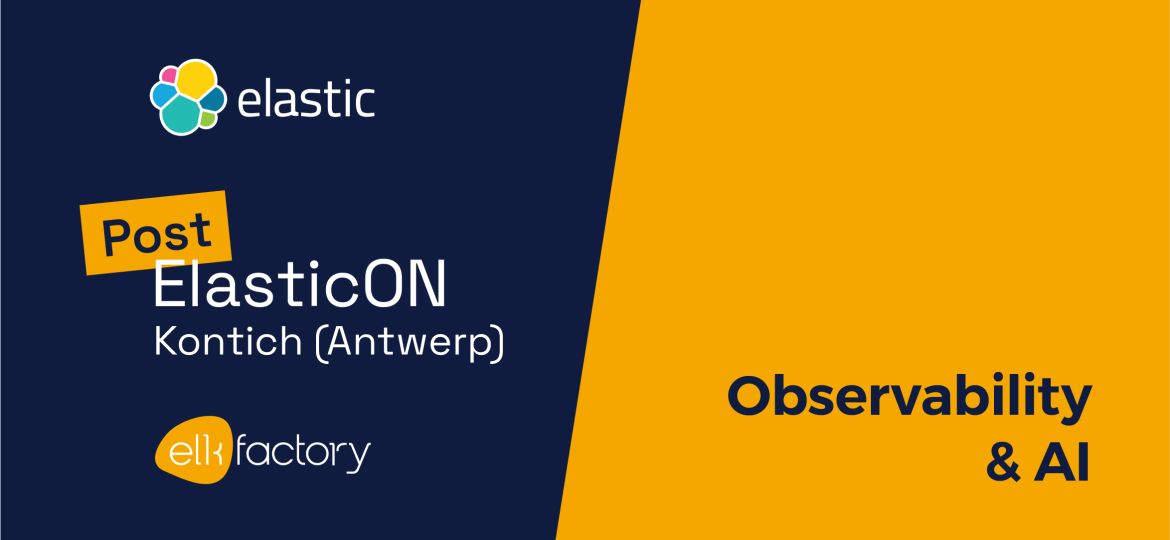
Post ElasticON: Observability and AI
The Evolution of Complexity
In the early days, when applications were monolithic and ran on a limited number of physical servers, manual processes and specialized tools sufficed for monitoring. However, the advent of cloud-native technologies ushered in greater efficiency alongside unprecedented complexity. A myriad of tools emerged to address the need for visibility, resulting in the sprawl of observability tools. We now find ourselves at another inflection point, grappling with an avalanche of data generated by a plethora of tools and technologies, fueled by the relentless growth of digitization.
Consider the monumental increase in applications, services, users, and customers over the past five years. This exponential growth has led to a data deluge, stored in siloed data stores across a distributed multi-cloud IT environment—a complexity never witnessed before. To tackle this challenge, teams now require AI-powered observability, not merely for unifying disparate data sources but for delivering actionable insights driven by the latest AI technologies, all at a petabyte scale.
Elastic Observability: Unifying Telemetry for Enhanced Efficiency
Enter Elastic Observability, a solution designed to unify all telemetry, whether business or operational. By ingesting high cardinality and high dimensionality data into a single scalable datastore powered by AI and analytics, Elastic breaks down silos, enabling context and correlation for faster root cause analysis. This full-stack observability solution provides interactive and context-aware insights, accelerating problem resolution and driving operational efficiency.

Harnessing the Power of AI: Elastic’s Extensive Capabilities
To consume and process large observability datasets at scale, Elastic offers extensive capabilities in AI and analytics. These include machine learning-based anomaly detection, automatic correlations for surfacing root causes of application errors and latency, and log categorization to streamline vast amounts of unstructured log data. Interactive chat-based investigations, driven by the Elasticsearch Relevance Engine (ESRE), empower users to make sense of complex data. With over 100 out-of-the-box open ML models, Elastic democratizes access to data and analytics across the entire organization.
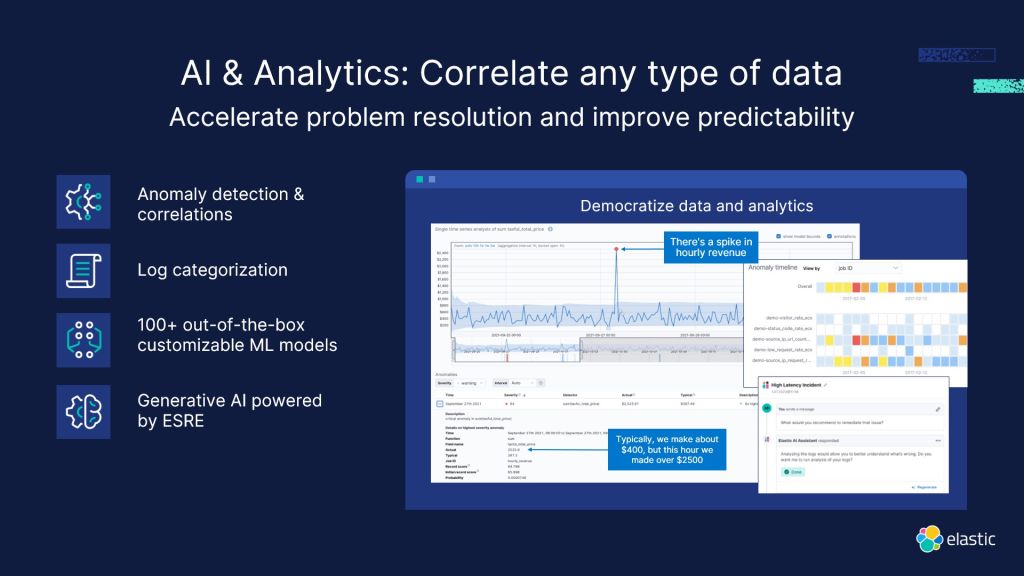
Elastic Observability and Machine Learning: Navigating Complexity
The integration of Elastic Observability with machine learning introduces innovative approaches to managing the challenges posed by complex digital environments. The zero-configuration, built-in machine learning facilitates AI-driven anomaly detection and root cause analysis across all observability data. This significantly reduces Mean Time to Detection (MTTD) and Mean Time to Resolution (MTTR), working seamlessly across thousands of dimensions.
Out-of-the-box anomaly detection for Application Performance Monitoring (APM) services and infrastructure monitoring, combined with ML jobs analyzing telemetry, allows teams to create alerts for high-scoring anomalies swiftly. AI-powered log categorization and anomaly detection further enhance the ability to make sense of billions of logs, identifying patterns and detecting changes in frequency.

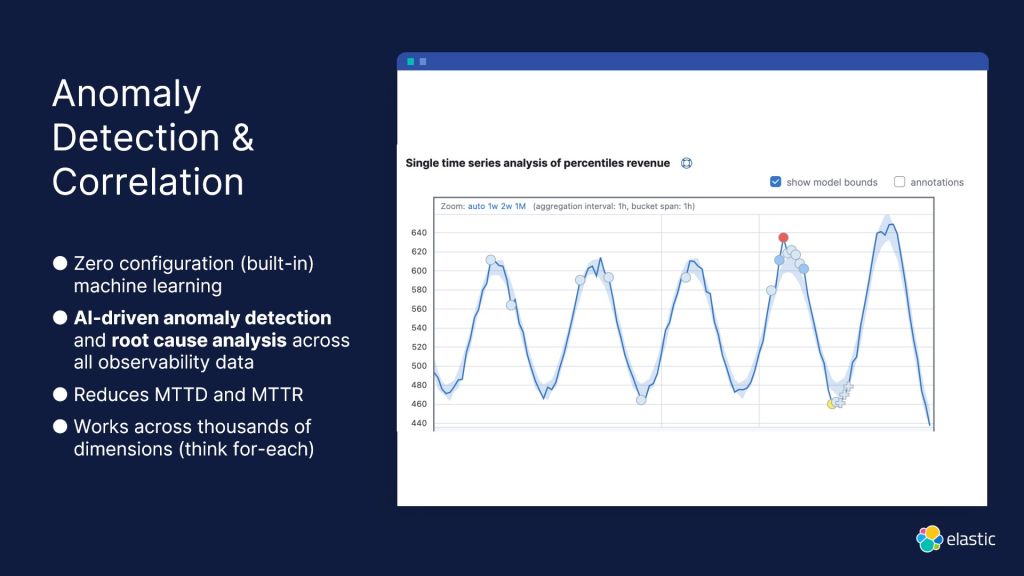
Elastic AI Assistant for Observability
To expedite incident management and root cause analysis, Elastic introduces an AI assistant. Powered by the Elasticsearch Relevance Engine and OpenAI, this assistant breaks down knowledge silos, allowing teams to interactively explore problems and execute remedies with generative AI. It generates context-aware, business-specific output based on proprietary data, such as knowledge bases and runbooks.
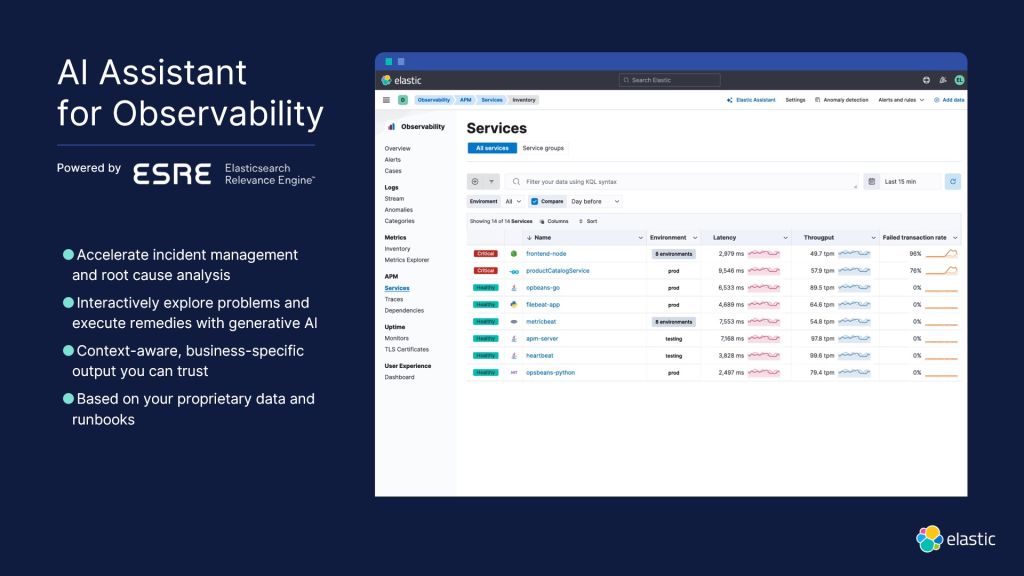
Shay’s Insights: The Power of Search and ESRE
Shay emphasizes the practicality and usefulness of Large Language Models (LLMs) in the context of Elastic Observability. The need for RAG (Relevance, Applicability, Grounding) is crucial, and Shay underscores why search technology, particularly Elastic, is the ideal solution. Elastic’s capabilities extend beyond being just a vector search engine—it excels in relevance, making it indispensable in the face of the data deluge.
Investments in Elastic’s Elasticsearch Relevance Engine (ESRE) further enhance its capabilities, combining vector search, broad search capabilities, and recent strides in context and relevance. Elastic emerges as not just the most downloaded or used search engine but also a formidable vector database.
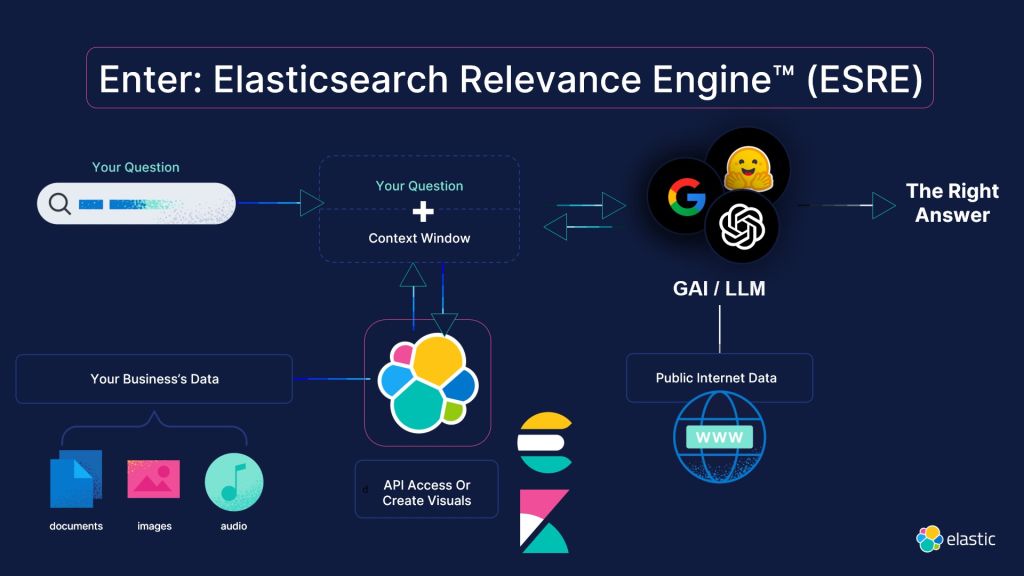
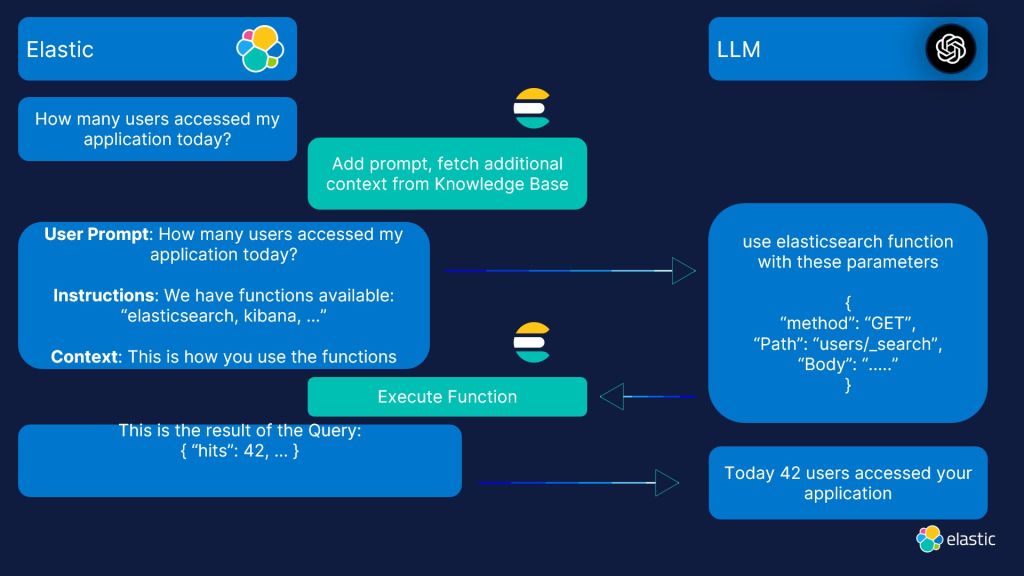
Challenges and Opportunities
Parsing through an overwhelming amount of information and data is a challenge that organizations face, and it’s only getting more complex. Data is the lifeblood of businesses, offering the potential for smart decision-making and improved operations. Elastic Observability, with its AI-powered capabilities, open and extensible platform, and unified contextual visibility, presents a tremendous opportunity for organizations.
In conclusion, Elastic Observability is not just a tool; it’s a comprehensive solution to the complex challenges posed by the modern digital landscape. With its advanced AI capabilities, it empowers teams to navigate the intricacies of data, providing actionable insights and driving operational efficiency in an era where data abundance is both a challenge and an opportunity.